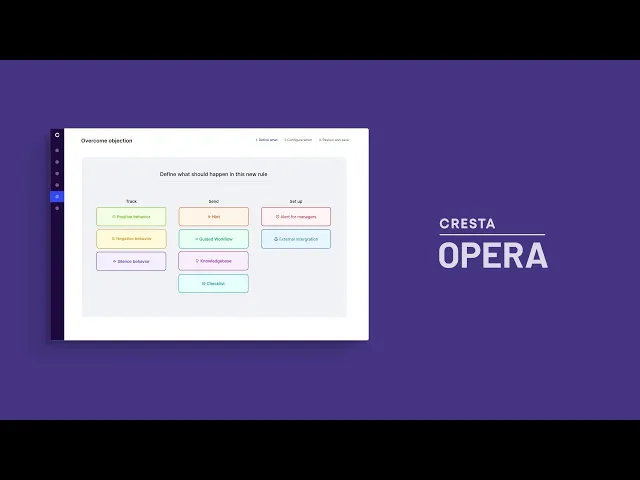
Gen AI for Opera: Democratizing AI Model Creation
No-code custom AI model development
When ChatGPT launched, our users wanted to experiment with AI to simplify their tasks. I partnered with the AI Delivery team to build a platform for developing and testing simple models. The key challenge was helping users understand AI's limitations and possibilities. I worked closely with engineering, AI specialists, and eager beta testers throughout development.
Vision
Empower users to build AI models independently, reducing internal team dependency and accelerating innovation.
Problem to be solved:
Enable customers to build language models without technical expertise.
Business need:
Reduce reliance on the internal team and expand self-service capabilities.
My role:
End-to-end design leadership and adoption strategy.
Outcome:
Beta launch attracted two new customers.
Learning:
Strategic hands-on guidance proved essential for successful adoption.
What is Opera?
Opera is Cresta's no-code platform to enable contact center leaders to automate coaching and monitor compliance without AI expertise.
It allows users to create custom "if this, then that" rules for simple scenarios, complementing Cresta's AI models that handle complex interactions.
Problem 1: Low accuracy
Keyword-only automation failed to accurately capture complex conversational moments.
Example: Detect when visitor is interested in new membership using keywords
Problem 2: Long wait
Users faced significant delays when requiring Cresta's AI team to build custom detection models.
Example: Detect when visitor is interested in new membership with a custom AI model
After extensive research and user interviews, we developed our first prototype and tested it with beta users. We simplified the process into three intuitive steps: 1️⃣ 2️⃣ 3️⃣ ... 🎩 🐰
In step 1 , user enters required information to train the model
In step 2, user marks what’s true or false to train the model
In step 3, user reviews the model predictions
What did we learn from the first iteration? 🤔
“I don’t speak this language”
Much of the technical jargon in product was foreign to end users.
"How do we ensure quality?
Users felt uncertain whether these simple steps were actually enough while the ML team wanted to keep simple but meaningful.
Solution 1: No need to speak the "AI lingo"
We eliminated AI terminology in favor of natural language. While this added some text, it greatly improved user's understanding of process.